Unlocking the Potential: The Role of Agriculture Datasets for Machine Learning
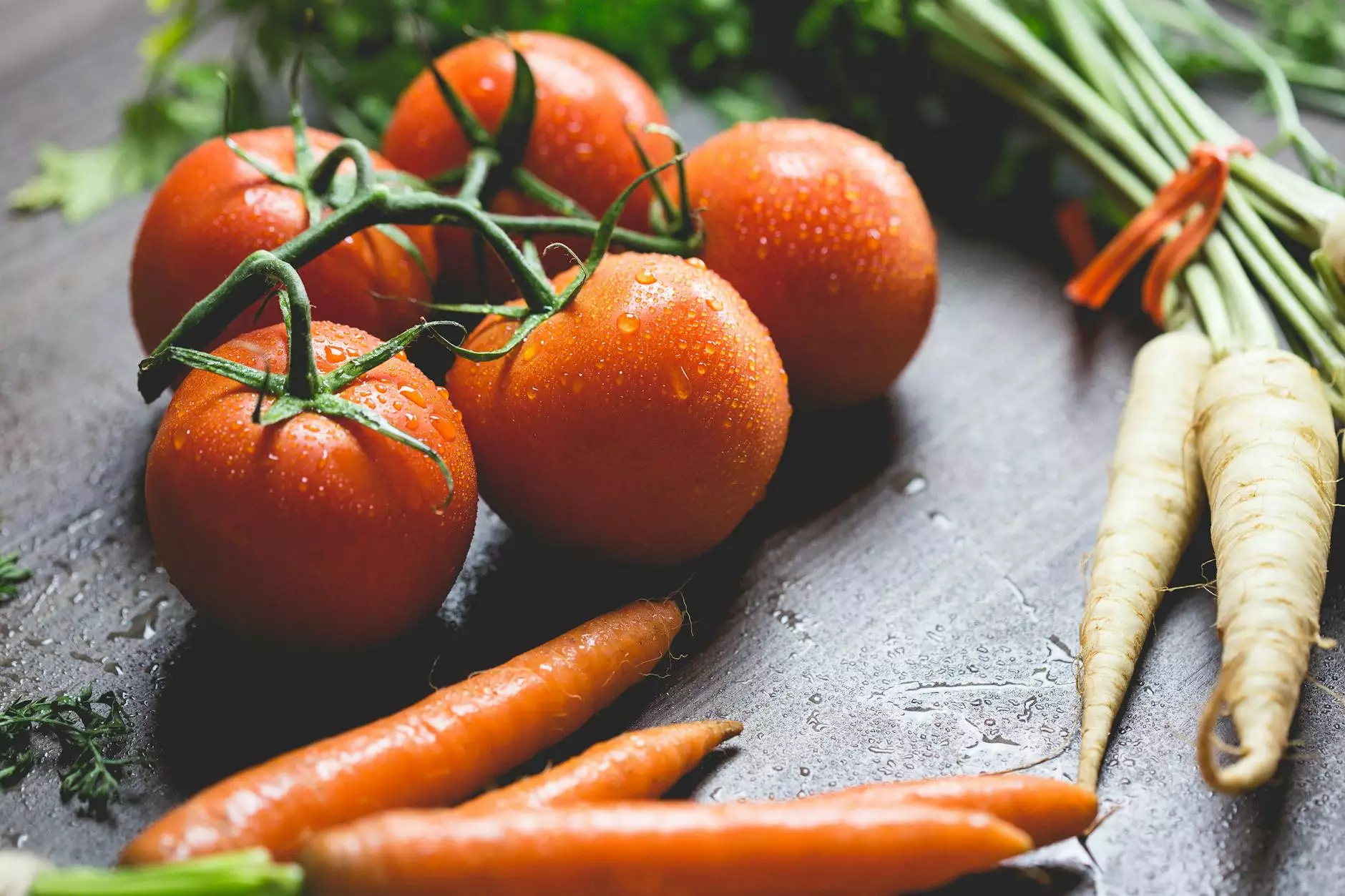
The agriculture dataset for machine learning has become a buzzword in the modern agricultural sector, marrying the fields of agriculture and technology. These datasets serve as the backbone for creating innovative machine learning models that can predict outcomes, optimize resource usage, and ultimately drive efficiency in farming practices.
1. Understanding Agriculture Datasets
Agriculture datasets can include a variety of information that ranges from soil characteristics, crop yield statistics, weather patterns, pest occurrences, and more. With academic institutions and organizations collecting vast amounts of this data, machine learning can tap into these datasets to unveil patterns and insights that were previously inaccessible.
2. The Intersection of Machine Learning and Agriculture
Machine learning involves training algorithms to make predictions or decisions based on data. In agriculture, this means utilizing agriculture datasets for machine learning to:
- Analyze Soil Health: Determining the best nutrient mix for enhancing crop production.
- Predict Weather Conditions: Informing farmers about impending weather challenges to reduce crop damage.
- Optimize Crop Rotation: Using historical data to identify the best crops to plant in succession.
- Manage Pest Control: Anticipating pest outbreaks through historical pest population data.
3. Why Datasets Matter in Agriculture
The importance of robust datasets in agriculture cannot be overstated. Effective agriculture datasets for machine learning help farmers and agronomists to:
- Make Data-Driven Decisions: Using predictive analytics, farmers can make informed choices that lead to higher yields.
- Reduce Costs: Identifying optimal planting times and resources can minimize waste.
- Enhance Sustainability: Sustainable practices can be promoted through the insights gained from data, leading to more environmentally friendly farming.
- Increase Food Security: Predicting crop yields and food shortages can help ensure a steady food supply.
4. Case Studies: Success Stories in Agriculture Using Machine Learning
4.1 Sustainable Farming Practices
One notable example involves a machine learning project focused on precision agriculture, which utilized agriculture datasets to improve irrigation systems. By analyzing data from soil moisture sensors, weather forecasts, and crop growth patterns, farmers were able to optimize water usage, thereby ensuring sustainable practices while improving crop yields.
4.2 Pest Prediction Models
Another successful application of agriculture datasets for machine learning was in the realm of pest control. By analyzing historical pest outbreak data alongside environmental variables, researchers developed predictive models that enabled farmers to apply pesticide at the right times, drastically reducing application frequency and costs while enhancing crop protection.
5. Key Components of Effective Agriculture Datasets
To fully utilize the power of machine learning in agriculture, it's essential to understand the key components that comprise effective agriculture datasets:
- Data Variety: Incorporating various data types, including satellite imagery, weather data, and soil telemetry, for a holistic view.
- Data Accuracy: Ensuring high-quality, accurate data is imperative for training reliable machine learning models.
- Timeliness: Data must be up-to-date to reflect real-time conditions affecting agricultural practices.
- Accessibility: Open access to datasets encourages collaboration and innovation within the agricultural community.
6. Challenges and Opportunities in Agriculture Data Utilization
As promising as the landscape appears for harnessing agricultural data, it is not without its challenges:
6.1 Data Privacy and Security
With an increasing reliance on data, concerns regarding data privacy and security have become prevalent. It is crucial for stakeholders to establish frameworks that protect sensitive information while leveraging available data for growth.
6.2 Integration of Diverse Datasets
Integrating diverse agricultural datasets can be complex but is necessary for creating comprehensive machine learning models. This requires significant effort in harmonizing different data formats and standards.
7. Future Trends in Agriculture Datasets and Machine Learning
Looking ahead, several trends are expected to shape the future of agriculture datasets and machine learning, including:
- Increased Use of IoT Devices: IoT technology will drive more real-time data collection from farms.
- Enhanced Predictive Analytics: Machine learning models will become more sophisticated, allowing for precise predictions.
- Collaboration Between Farmers and Researchers: Building partnerships will facilitate better data-sharing practices and empower innovation.
- Focus on Sustainable Practices: Machine learning insights will aid in the design of practices that promote sustainability.
8. Conclusion: The Future of Agriculture is Data-Driven
The integration of agriculture datasets for machine learning is transforming the agricultural landscape, enabling farmers to make data-driven decisions that promote productivity and sustainability. As technology continues to advance and datasets become richer and more comprehensive, the potential for innovation in agriculture is boundless. By harnessing the power of data, the agricultural sector can not only increase yield but also contribute to a more sustainable future.